Tanya Berger-Wolf never thought she would become an environmentalist. She fell in love with math at a young age and pursued a doctorate in computer science, gaining attention for her advanced theoretical research. However, just as she was about to graduate, she became captivated by a surprising topic: zebras.
While still an undergraduate, Berger-Wolf started working as a research assistant in the ecology department, creating computer simulations of wildlife populations. She was fascinated by the fact that digital technologies and biodiversity were developing at different rates. While the digital sector was growing rapidly, populations of endangered species were declining. Unlike the abundance of data in computer science, Berger-Wolf was astonished by the scarcity of data on the world’s most endangered species.
The animal that drew Berger-Wolf’s attention was the Grevy’s zebra, historically known as the imperial zebra. Once roving in large herds over vast areas of East Africa, these largest of the wild equines, noted for their elegant stripes and remarkable gait, now number fewer than 1,000. They are being displaced by farming and cattle ranges, and are still hunted for their skins and meat. When Berger-Wolf learned about their plight, scientists were predicting that this iconic species might disappear within two decades.
How, exactly, could computer science help save endangered species?
Berger-Wolf considered whether she could use her digital skills to aid in saving the zebras.
However, the computer scientists she consulted discouraged her. One told her, “You’re smart enough to do theory; why do you want to do this applied crap?” Others suggested that she switch to computational biology. Berger-Wolf tried it, only to realize that she preferred to focus on something that combined ecology and computer science to help protect the planet.
The area of research she envisioned did not have a name or precedent. Nevertheless, Berger-Wolf inquired about the best ecologist in the world and was directed to Simon Levin at Princeton University. She proposed to Levin an unconventional idea: to create a new field called computational ecology—an ambitious research agenda like computational biology, but focused on ecological issues. Levin accepted, and Berger-Wolf moved to Princeton.
Even at Princeton, she faced challenges. Most ecologists viewed computer scientists as mere coders, and most computer scientists saw ecologists as just sources of data. Berger-Wolf struggled to convince her fellow computer scientists of the potential of her vision. While the ecologists were intrigued, they were skeptical about how computer science could help save endangered species.
ZZebras were Berger-Wolf’s breakthrough. Shortly after arriving at Princeton, she discussed the issue with Daniel Rubenstein, a leading behavioral ecologist. She explained the problem: Ecologists wanted the Kenyan government to implement stronger protections, including a national park, but the government demanded an accurate census before proceeding with regulations that would likely face strong resistance from local communities.
Why didn’t I think of it before? All we need is a bar code reader for zebras!
In this situation, ecologists were stuck. The usual ways of counting zebras (like catching them, marking them, or using anesthesia to attach tracking devices) were expensive, traumatic, and put the zebras at risk of getting sick. Because the zebras were so endangered, they couldn't use these normal ways. But looking at the zebras and counting them was slow, costly, and not very accurate; it could take up to thirty minutes to spot a zebra in a photo, and the zebras were really hard to find. The baby zebras in the group were dying a lot, but without a count, the government wouldn't help. If nothing changed, the zebras were in big trouble.
How could computer science help? Berger-Wolf had a realization while teaching a course about computational ecology in Kenya. She had already met and married her husband—someone who studied zebras. One evening, Berger-Wolf heard her husband and some other field biologists talking about how to identify individual zebras from photos, which was a boring job that would take many days. They thought that they just needed a way to automatically identify and list the zebras. It had been another frustrating day trying to find the hard-to-spot animals, and Berger-Wolf heard her husband say: “Why didn’t I think of it before? All we need is a bar code reader for zebras!”
Berger-Wolf suddenly understood. Zebras do have unique stripe patterns. While a bar code reader might not work, something like a fingerprint scanner could potentially work.
In theory, zebra stripes are similar to human fingerprints, in that each zebra has its own unique patterns. While biologists had long used these patterns (which they sometimes call “bodyprints”) to help identify individual zebras in the wild, they had never automated the process.
Berger-Wolf and her Ph.D. student Mayank Lahiri started working together to create an initial version: StripeSpotter. Their aim was to make a free program that anyone could use to upload a photo of a zebra’s side, for automatic identification. The process is simple: The zebra is assigned a “stripe code,” which is then checked against the database. If the zebra is already in the database, it is matched to earlier photos; if not, it is given a new, unique identity. The AI-driven program can identify individual zebras from a basic photo and can handle images of different sizes and angles, and photos with different lighting. After testing it with thousands of zebra photos, including hundreds she took during airplane flights over northern Kenya, Berger-Wolf confirmed that the algorithm was accurate. With enough photos, a full count of the zebra population was now possible.
Berger-Wolf then joined forces with Chuck Stewart, a computer scientist at Rensselaer Polytechnic, and his student Jon Crall, to create a more thorough computer vision method for identifying patterned animals, which they named HotSpotter. The once nearly impossible job of identifying and tracking individual animals, which used to take weeks or months, now takes just milliseconds. Just as dependable as facial recognition technology, computers can now recognize zebras as individuals.
Berger-Wolf knew her idea could work for many of the almost 9 million known species on the planet. Also, photos could be added from satellites, drones, and social media, making it a low-cost face recognition reader for wildlife. The only thing she needed was a good data management system.
Berger-Wolf thought of StripeSpotter around the same time physicist Zaven Arzoumanian became interested in endangered whale sharks.
Whale sharks are unknown to a great extent, but their global populations have decreased by half in recent decades. Arzoumanian's interest grew when his friend Jason Holmberg, a software programmer, had a special encounter with a whale shark while scuba diving. Holmberg wondered how to track the elusive fish and sought Arzoumanian's help at NASA's Goddard Space Center.
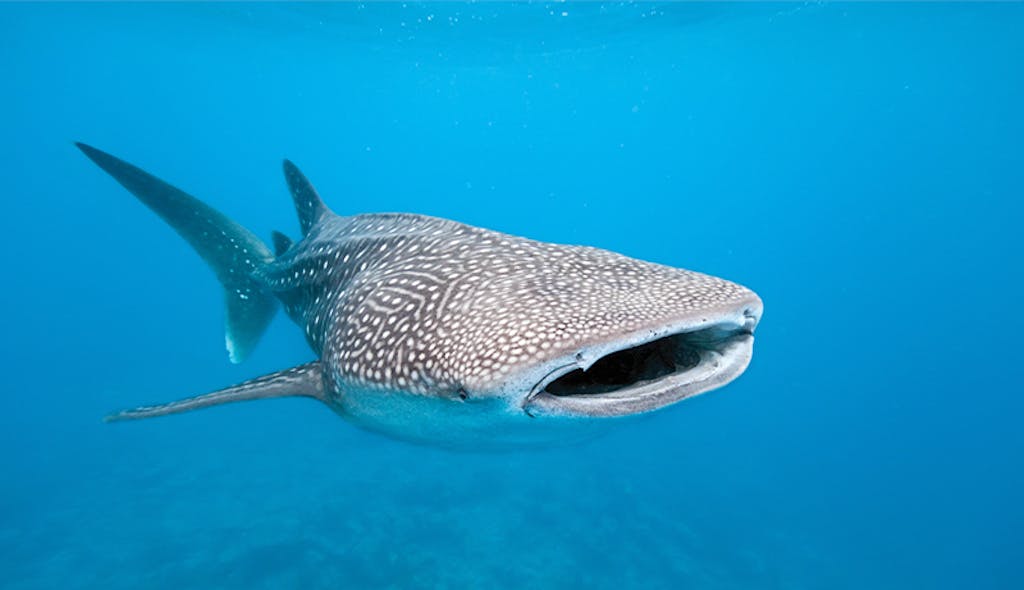
How could they automate the identification of whale sharks? The creatures have hundreds or thousands of distinctive white spots on their backs, like fingerprints, but it's hard for human eyes to distinguish.
Arzoumanian found a stellar pattern-matching algorithm that astrophysicists developed decades ago to analyze the stars revealed by the Hubble Space Telescope. This algorithm could be repurposed to identify individual whale sharks based on their unique skin patterns.
The universe contains 30 billion trillion to 70 billion trillion stars, overwhelming astronomers with information. Groth's algorithm solved this, and Arzoumanian realized it could be used to identify individual whale sharks, whose dappled skins have unique patterns as intricate and unique as stellar constellations.1
Holmberg and Arzoumanian adjusted the algorithm and collaborated with whale shark biologist Brad Norman. Norman's network of amateur whale shark spotters uploaded photographs, and the algorithm identified individual sharks in seconds.
Norman previously identified whale sharks by eye, which was a tiring process. With the automated method, over 6,000 individual whale sharks were identified, with a successful match rate of over 90 percent, thanks to the contributions of more than 5,000 citizen scientists who reported tens of thousands of whale shark encounters in dozens of countries.
The amount of known places where whale sharks gather has doubled in just a few years. This discovery would not have been possible using manual observation methods.2 According to Norman, whale sharks are no longer seen as random animals, but as individuals with their own stories, histories, and futures yet to be written. This makes it very attractive as a citizen science project.3
By involving over 8,000 citizen scientists and using YouTube videos, researchers found more than 12,000 individual whale sharks from over 75,000 reported sightings. These findings led the International Union for Conservation of Nature (IUCN) Red List of Threatened Species to reclassify the whale shark from vulnerable to endangered. It was also determined that the population trend was declining, rather than stable as previously thought.
BAfter their successful experience with whale sharks, Arzoumanian and Jason Holmberg established a non-profit organization called Wild Me. However, they faced a challenge: their original algorithm, StripeSpotter, couldn't handle the scale. Fortunately, Holmberg had developed a strong data management layer, which was exactly what Berger-Wolf needed. The three innovators teamed up and replaced the constellation and StripeSpotter algorithms with the HotSpotter-based approach, connected to the Wild Me data management layer. This created a system that could potentially catalog any living thing, as long as it had colors, stripes, spots, wrinkles, scars, or notch patterns that do not change with age.
They then set out on an ambitious mission to catalog the world’s wildlife. There was a great demand for automated animal identification systems. Berger-Wolf was inundated with requests. Researchers submitted large sets of photos from around the world. The vast amount of data now available was both a blessing and a curse: the Wild Me team had a huge cataloging issue, and it still wasn't clear how conservationists could use the information. At this point, the team had attracted half a dozen engineers and created Wildbook, a version of Facebook for animals. The founders had a grand vision: blend wildlife research with citizen science and computer vision to speed up zebra monitoring. They presented it as software that combats extinction. Their ultimate aim was to create a universal animal recognition algorithm that could identify unique individuals in every species on the planet, like a low-cost facial recognition reader for the world’s wildlife. With such an algorithm, ecologists would be able to easily and automatically identify and track any individual living creature on the planet, from birth to death..
Several years later, Wildbook covers hundreds of species. If you’re interested in whales or dolphins, you can check out Flukebook. The visible part of the website is the social media side, where the public can visit and possibly become attached to some charismatic megafauna or microfauna. Behind the scenes, a set of algorithms use computer vision and citizen science to give a face, name, and story to every single animal on the planet.
Years later, Wildbook includes hundreds of species. If you’re a fan of whales or dolphins, you can visit Flukebook. The visible part of the website is like social media, where the public can visit and maybe form an attachment to some charismatic megafauna or microfauna. In the background, a set of algorithms utilize computer vision and citizen science to give a face, name, and story to every single animal on the planet.
Whale sharks stopped being random animals and became individuals with stories and futures.
The platform is now being used to keep track of a Noah’s ark-like list, such as whales, giraffes, manta rays, humpback whales, Hector’s dolphins, sea bass, flapper skates, turtles, sharks, jaguars, lynx, seals, polar bears, and sea dragons. With support from the Moore Foundation, Berger-Wolf is expanding Wildbook to thousands of species. In the future, she hopes to create organized evaluations of populations for every species on the IUCN Red List, a global catalog of endangered species.
Another supporter of Wildbook has been Microsoft’s AI for Earth program. Why did Microsoft decide to get involved? In the words of Josh Henretig, who managed the $50 million fund Microsoft is investing in AI for Earth: “What’s really worrying to us and to many scientists around the world is that we have only discovered/described about 1.5 million species of an estimated 10 million on our planet, and less than 5 percent of that 1.5 million species have ever been analyzed in any detail. There are species that are disappearing off our planet that we’ve never even known about.”
Many researchers are now emulating Wildbook’s methods, developing machine learning algorithms to monitor specific species of wildlife (chimps, dolphins, badgers, birds, koalas, kangaroos), and even track the exotic pet trade. These efforts are not enough to halt extinction, but they are one important piece in the larger puzzle: accurate documentation of species decline.
In the meantime, the Grevy’s zebras now have a fighting chance at survival.
Soon after the launch of HotSpotter, Dan Rubenstein came up with a controversial suggestion: persuade the Kenya Wildlife Service to incorporate HotSpotter into a biannual zebra population census. As Berger-Wolf remembers, “The computer scientists on our team nearly had a heart attack when Dan proposed a census.” But Rubinstein insisted. They needed to convince the government to do a census, and they also needed to win over the public by engaging ordinary Kenyans in the process.
Although doubtful, Berger-Wolf approached the Kenyan government, and to her surprise found some interest. After two years of negotiations and planning, the first two-day Great Grevy’s Rally was held across Kenya in 2016. The rally was portrayed as a blend of citizen science and public relations, mobilizing hundreds of Kenyans, from the prime minister to children from the slums of Kibera, to take photos of the elusive zebras in a two-day national campaign. The rally produced an unprecedented result: a verifiable, accurate census (rather than estimate) of the entire Grevy’s population.
Over subsequent years, its accuracy was confirmed; meanwhile, the political popularity of the zebra grew. As Berger-Wolf recounts, the youngest participant in the most recent rally was 3 years old, while the oldest was over 90 years old. The Kenyan government was able to enact reforms that were previously thought to be too unpopular to be feasible: passing a new Grevy’s Zebra Endangered Species Management Plan (committing land, resources, and funding) and strategically limiting lion populations (a major zebra predator) with contraception.
A government survey in 2020 found that, for the first time in a while, the population of Grevy’s zebras in Kenya had stopped decreasing and stayed about the same.
This excerpt is taken from Gaia’s Web: How Digital Environmentalism Can Combat Climate Change, Restore Biodiversity, Cultivate Empathy, and Regenerate the Earth by Karen Bakker, published with permission from The MIT Press.
Lead image: slowmotiongli / Shutterstock
References
1. Arzoumanian, Z., Holmberg, J., & Norman, B. An astronomical pattern-matching algorithm for computer-aided identification of whale sharks Rhincodon typus. Journal of Applied Ecology 42, 999–1011 (2005).
2. Norman, B.M. et al., Undersea constellations: The global biology of an endangered marine megavertebrate further informed through citizen science. BioScience 67, 1029–1043 (2017).
3. Annie Sneed, A. Astronomy Tool Helps ID Sharks. Scientific American ( 2018).